Statistical Drift Monitoring in Pyranometers: How to Ensure Reliable Data in Solar Plants
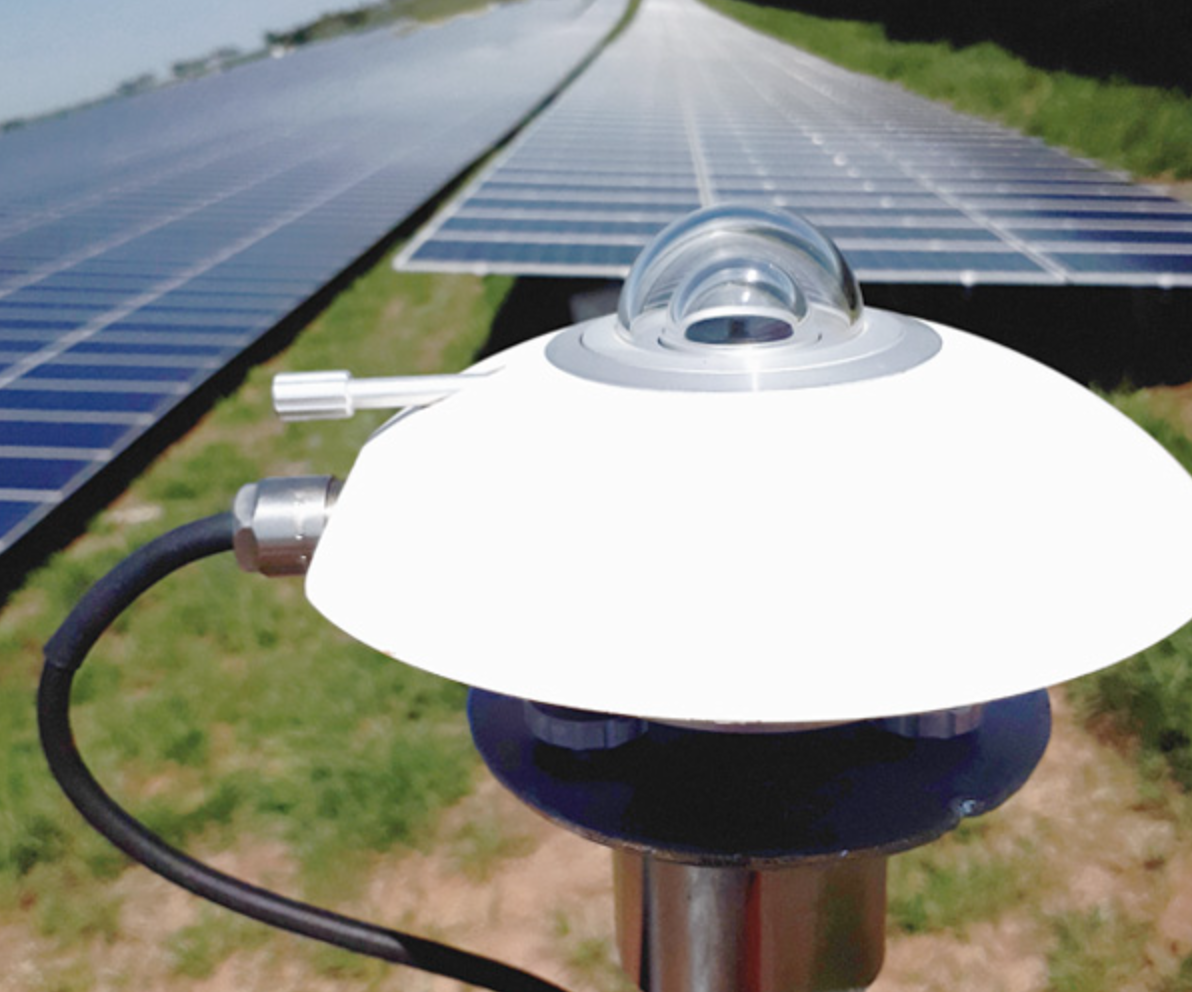
For a photovoltaic solar plant to operate efficiently and reliably, accurate solar radiation measurement is crucial. These data are used to evaluate equipment performance and inform operational and maintenance (O&M) decisions. However, pyranometers—sensors that capture solar radiation—can naturally drift up to ±2% per year. In adverse environmental conditions, this deviation can be even greater, compromising performance analysis.
The Delfos Energy technical team developed and tested two innovative statistical methodologies to automatically monitor pyranometer drift. The results of this study were published at EU PVSEC (European Photovoltaic Solar Energy Conference and Exhibition) and can deliver significant benefits for professionals in solar O&M. Let’s explore the findings.
The Challenge: Detect Drift Before It Becomes a Problem
Pyranometers must be periodically calibrated to ensure accuracy. However, factors such as high temperatures, dirt, and aging can cause premature data deviations. Early detection of such deviations prevents energy loss and optimizes maintenance costs.
To address this, Delfos developed two approaches that compare neighboring sensors within the same meteorological station to “watch” each other.
Methodology 1: Simple and Straightforward with Confidence Bands
This method is based on a logical premise: identical sensors under the same conditions should show very similar readings. Delfos calculated the daily difference between each sensor and the average of two neighboring sensors at four solar plants in Brazil using 30 months of historical data.
During the “healthy” period (right after calibration), these differences formed a statistical pattern. The Interquartile Range (IQR) method was used to define acceptable upper and lower limits. Whenever a sensor’s reading exceeded these bounds, an automatic alert was generated.
Result: This approach worked well, especially during high irradiance periods when sensors experience more thermal stress.
Limitation: Alerts decreased during low irradiance periods, allowing subtle deviations to go undetected.
Methodology 2: Refined Approach with Segmentation and Gradual Alerts
To overcome the above limitation, a second, more sophisticated methodology was created. It segments data by irradiance levels (low, medium, high, very high) and introduces two alert levels: "warning" and "alarm."
A moving 7-day monitoring window was also implemented to smooth temporary fluctuations and reveal trends.
Result: This method generated consistent alerts even during low solar seasons, making it a more robust tool for O&M teams.
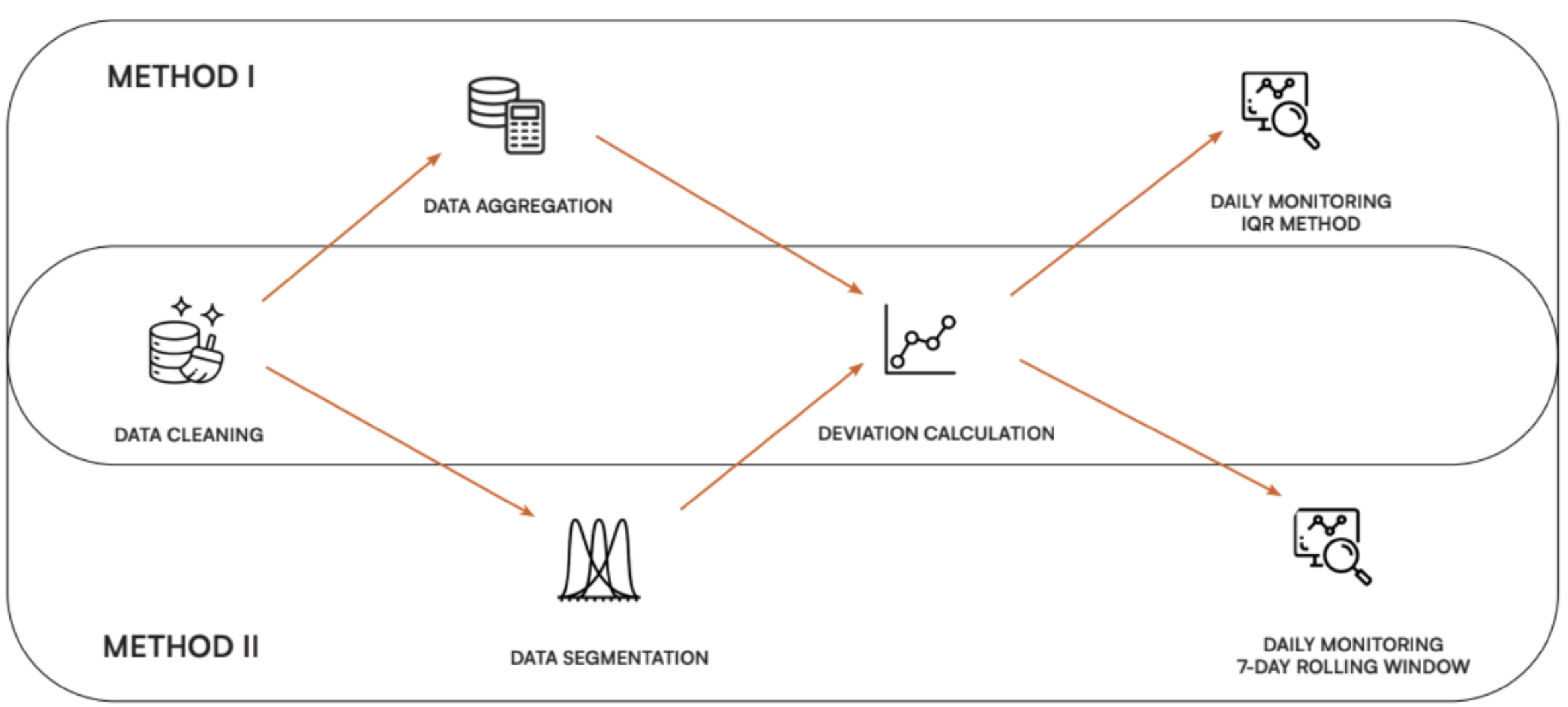
Conclusion: Practical Benefits
Implementing these methodologies in daily monitoring allows O&M teams to be quickly notified of any sensor behaving abnormally. This means:
- More focused and efficient inspections
- Reduced costs from unplanned corrective maintenance
- Increased reliability of solar radiation data for performance evaluation and revenue assurance
Delfos also plans to explore machine learning techniques (e.g., Linear Regression, XGBoost) to further enhance alert consistency, especially considering radiation seasonality.
About the Authors and Publication
Study by Delfos Energy specialists Lucas T. Silva, Rodrigo S. Queiroz, Nathianne M. Andrade, and Danielle B. Cavalcante. Presented at EU PVSEC 2024, one of the world's leading solar energy events.
To access the full study and rich content, fill out the form below:
Book a meeting
Let's connect and forge new partnerships
Custom Renewable Energy Solutions
Contact us today to discuss your renewable energy needs and find the perfect solution for your business.