The future of distributed generation: How artificial intelligence is revolutionizing the efficiency of solar plants

The energy transition is already a reality, and solar distributed generation is at the center of this transformation. But did you know that artificial intelligence (AI) is increasing the potential of solar plants? With technological advancements, predictive models, machine learning, and real-time analytics are optimizing energy production, reducing operational costs, and ensuring greater system reliability.
In this article, we will explore how AI is revolutionizing the distributed generation sector and what to expect in the future.
What is solar distributed generation?
Distributed generation (DG) refers to the production of electricity close to or at the point of consumption, often through photovoltaic solar panels. This model promotes greater energy autonomy, cost reduction on electricity bills, and lower environmental impact, in addition to contributing to the decentralization of the power system.
According to the Brazilian Electricity Regulatory Agency (ANEEL), Brazil surpassed 35 gigawatts (GW) of installed capacity in micro and mini distributed generation by January 2025, with more than 3 million systems connected to the national power grid.
According to data from the International Energy Agency (IEA), the global installed capacity of photovoltaic solar energy reached 879 gigawatts (GW) in distributed generation systems in 2024, with 537 GW in residential applications and 522 GW in commercial and industrial (C&I) systems.
This growth reflects the increasing role of distributed generation in the global energy transition. The decentralization of the energy matrix, driven by digitalization, regulatory incentives, and the adoption of technologies such as artificial intelligence, has enabled greater energy autonomy for consumers and more operational efficiency for integrators and operato
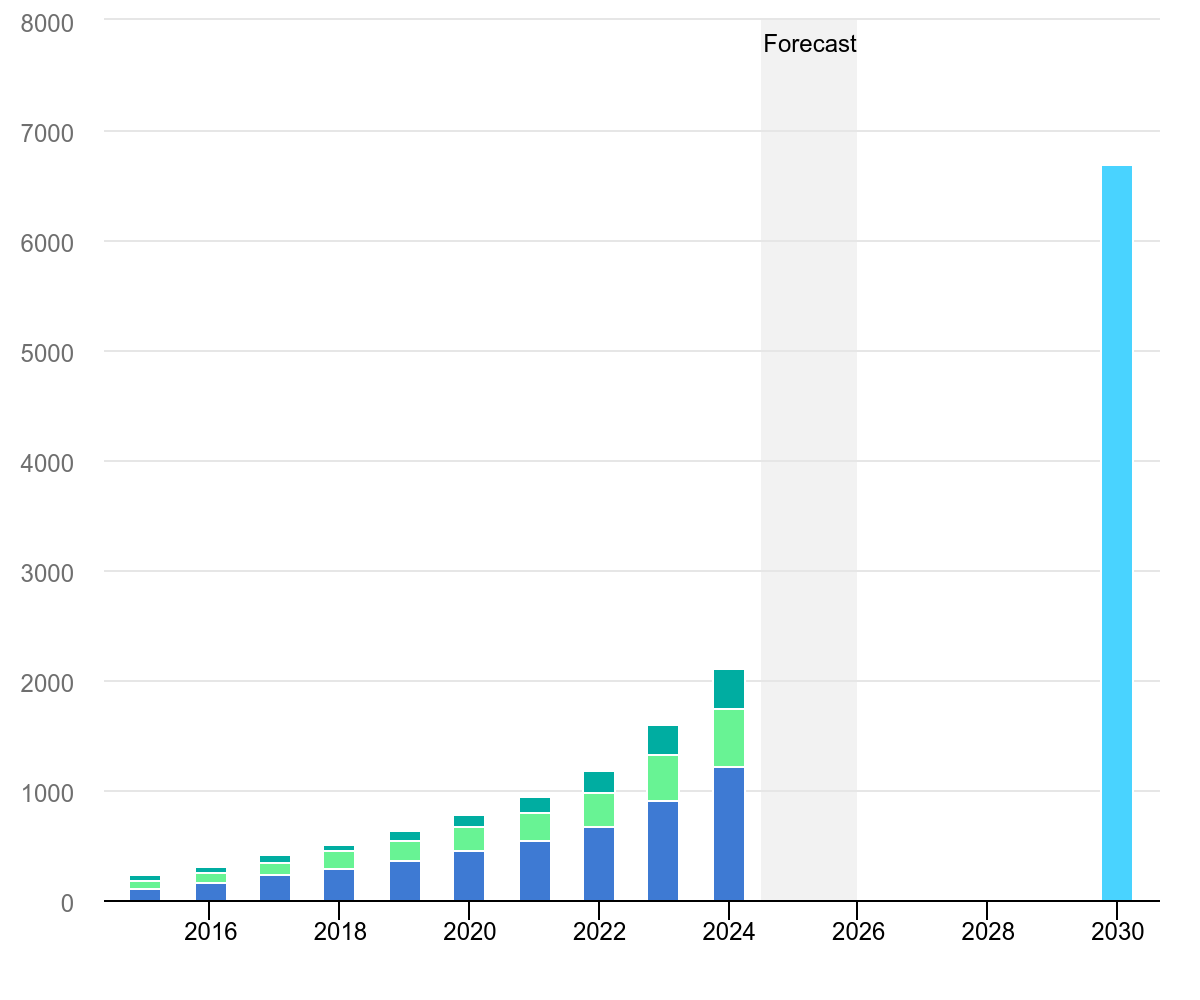

Challenges of efficiency in solar plants
Despite its popularity, DG faces technical and operational challenges such as:
- Intermittency caused by climate variations
- Reactive maintenance, which can generate losses
- Difficulty in large-scale monitoring
- Management of multiple distributed assets
This is where artificial intelligence becomes a game-changer.
How artificial intelligence is transforming the sector
1. Failure prediction and predictive maintenance
AI can identify abnormal patterns in equipment performance before they fail, allowing for proactive actions. According to a McKinsey study, predictive maintenance can reduce machine downtime by 30% to 50% and increase their lifespan by 20% to 40%.
2. Optimization of energy generation
Optimizing solar energy generation is one of the most effective applications of artificial intelligence in the energy sector. Through machine learning algorithms trained with historical production data, weather information, and operational parameters of the equipment, it is possible to make highly accurate photovoltaic generation forecasts over different time horizons, from minutes to days.
This accurate forecasting is essential for solar plants operating in the free energy market, where the reliability of delivering contracted energy directly impacts profitability and avoids regulatory penalties. Furthermore, AI allows for optimizing energy dispatch in hybrid systems, such as those combining solar with batteries or other intermittent sources, generating greater added value.
According to academic studies and applications in the renewable energy sector, using AI- and machine learning-based predictive models can significantly improve solar forecasting accuracy. Research such as that conducted by UNESP has shown that machine learning models, such as artificial neural networks and support vector regression, outperform traditional forecasting methods based solely on historical weather data, especially in environments with high climate variability.
Moreover, AI-based solutions enable dynamic operational adjustments, identifying in real-time the best moments to invert production, manage solar trackers, and prioritize more efficient areas of the solar field, reducing losses and maximizing return on investment.
A concrete example is the use of platforms like Google DeepMind, which applied machine learning algorithms to forecast wind farm energy production up to 36 hours in advance. This predictability allowed for better integration of renewable sources into the power grid and generated a 20% increase in the economic value of the supplied energy by better aligning supply with grid demand.
3. Automatic detection of dirt and shading
The efficiency of a solar plant is directly related to the intensity of irradiation that reaches the photovoltaic modules. However, factors such as dust accumulation, organic debris, air pollution, or partial shading from objects, vegetation, or nearby structures can significantly compromise system performance, causing losses in total energy generation.
With the use of computer vision integrated with artificial intelligence models, it becomes possible to automatically and in real-time detect hotspots, soiling, or shaded areas. These anomalies, previously identified only through manual inspections or periodic thermographies, can now be recognized through thermal cameras, autonomous drones, or embedded sensors connected to the cloud.
Platforms like Delfos apply proprietary algorithms to correlate performance data with field images and measurements, allowing for granular performance analysis by plant, inverter, or string. The system precisely indicates where losses occur and recommends targeted interventions, such as selective cleaning or strategic pruning, eliminating unnecessary preventive maintenance and reducing operational costs.
This data-driven approach ensures that O&M (Operation and Maintenance) resources are used intelligently, increasing asset availability and ensuring higher financial returns for operators. Additionally, traceability of occurrences allows for quicker action, mitigating risks of accelerated degradation and optimizing equipment lifespan.
4. Intelligent portfolio management
As the number of solar plants grows, especially in the context of distributed generation and large operators with dispersed assets, it becomes essential to have tools that offer centralized, scalable, and intelligent portfolio management. This is where artificial intelligence stands out as a strategic ally.
AI-based platforms, such as those developed by Delfos, enable the simultaneous monitoring of hundreds of distributed assets in different locations, consolidating operational data in integrated and intuitive dashboards. These systems apply algorithms that continuously assess the performance of each asset, classifying occurrences by criticality, financial impact, and urgency of resolution.
This allows the O&M manager to prioritize interventions based on ROI, visualize comparisons between plants, detect patterns of systemic inefficiency, and generate automated reports with strategic insights. AI also facilitates the creation of multisite predictive models that learn from the behavior of one plant to anticipate failures in others with similar characteristics, a valuable resource in heterogeneous portfolios.
Furthermore, this approach drastically reduces the reliance on manual and reactive analysis, providing agility, precision, and governance to decision-making processes. Intelligent portfolio management turns solar energy operation into a data-driven journey where each asset is continuously optimized to deliver its maximum potential.
Real case: artificial intelligence applied to renewable generation
Delfos has been transforming renewable energy asset management with the practical application of artificial intelligence. A clear example is the project developed in partnership with V2i Energia, a company that operates globally in the management of wind farms, hydroelectric plants, and transmission lines.
Despite high availability rates, the wind farm monitored by V2i experienced short and recurrent drops in production, hindering the full utilization of its potential. Delfos, together with the O&M team and V2i, implemented a strategy based on predictive models and remote operational intelligence capable of identifying critical turbines, pointing out probable causes of failures, and guiding more precise maintenance actions.
The result was significant:
- 18% reduction in energy impact caused by unavailability
- +174 MWh of energy produced in just 4 months
- Better field activity targeting based on real data and smart alerts
This case demonstrates how integrating AI into diagnostics and maintenance planning processes directly contributes to reducing costs, increasing production, and improving operational efficiency of renewable assets.
The future: integration with the grid and carbon market
As the global electricity sector moves towards decentralization, digitalization, and decarbonization, artificial intelligence is becoming a key element for the full integration of distributed generation into smart grids. This transformation demands a more responsive, interconnected, and data-driven energy ecosystem.
AI enables DG systems to operate in coordination with the grid through features such as automated demand response, dynamic surplus trading in the energy market, and predictive storage management with batteries. Additionally, integration with emerging technologies such as blockchain enables tokenization and tracking of carbon credits, ensuring transparency, auditing, and environmental valuation of renewable assets.
With the convergence of AI, IoT, and distributed energy models, the future points to a cleaner, more efficient, and resilient energy system, where each solar plant, even small-scale, becomes a strategic piece in the stability and sustainability of the power grid.
Conclusion
Artificial intelligence is shaping, in real-time, the future of solar distributed generation. By enabling data-driven decisions, anticipating failures, maximizing production, and intelligently integrating multiple assets, AI delivers not only operational efficiency but also strategic intelligence to the sector.
In a scenario of rapid solar matrix expansion and increasing competition among operators, plants that adopt advanced monitoring and prediction solutions, such as those developed by Delfos, will be positioned to lead this new phase of the energy transition with more performance, more profit, and a greater positive impact on the planet.
Book a meeting
Let's connect and forge new partnerships
Custom Renewable Energy Solutions
Contact us today to discuss your renewable energy needs and find the perfect solution for your business.