How EDiGaPS is Revolutionizing the Way We Understand the Impact of Turbulence on Wind Turbines

The Hidden Costly Problem
Wind turbines operate under various wind conditions but are often tested in sites with different wind profiles. This complicates performance monitoring. For accurate comparisons, adjustments for factors such as air density and turbulence are necessary. However, turbulence is often overlooked.
Turbines are installed in regions with varying wind conditions, but current methods — such as those in IEC 61400 — only partially address the issue: correcting for the bias caused by the 10-minute average. This accounts for only about 50% of the actual turbulence effects, according to Bardal & Sætran (2017).
How to apply a more comprehensive correction?
Delfos’ Approach: Better Corrections Using Real Data
EDiGaPS (Equivalent Difference in Gaussian Process Surface) is Delfos' response to this challenge. Developed by the Data Science team, the model uses Machine Learning with Gaussian Processes to build a three-dimensional surface (wind speed x turbulence intensity x power) based on real SCADA data.
The idea is simple but powerful: instead of applying a generic formula, EDiGaPS learns from the turbine's own data how turbulence affects production and corrects the power curve accordingly.
What Was Tested?
- Training: 7 turbines (1.8 MW), 7 months of SCADA data
- Test: 1 turbine, 15 months of data
- Scenarios Analyzed: low (10%), medium (16%), and high (22%) turbulence
- Success Metric: how well the method eliminates AEP (Annual Energy Production) differences between scenarios that should be practically identical aside from turbulence differences
And the Results?
Compared to the traditional IEC method:
- EDiGaPS corrected an average of 60.2% of the turbulence effects
- Avoided overcorrections, which occurred in the IEC normalization and can lead to unrealistic production forecasts
- In some cases, reached close to 100% correction of AEP differences caused by turbulence
In summary: it corrected better and more balanced.
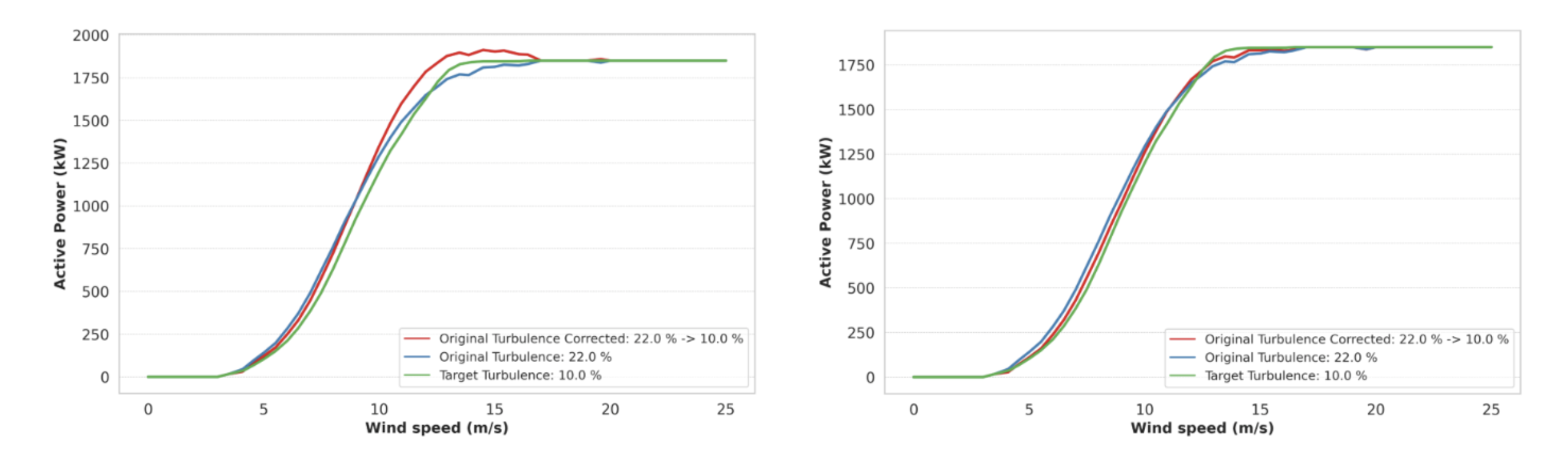
Why Does This Matter?
For those managing wind farms, this means:
- More accurate performance assessments
- More reliable decisions regarding O&M and warranty
- Less uncertainty in AEP simulations for investors
For the sector as a whole, it represents a major step toward more realistic and customized models, tailored to each site's unique characteristics.
What’s Next?
EDiGaPS has already demonstrated its value on one turbine type. The next steps include:
- Testing on different models and manufacturers
- Evaluation across varied wind regimes
- Expanding collaborations with operators and manufacturers for large-scale trials
Conclusion
EDiGaPS is a clear example of how data and machine learning can drive tangible improvements in the way we analyze and optimize renewable assets.
This work underscores Delfos’ mission to lead in predictive maintenance and applied intelligence for renewable energy — turning science into action.
About the Authors and Publication
Based on the paper presented at Wind Europe 2025.
This study was conducted by Fernando Saraiva Filho, Nathianne Andrade, Rodrigo Queiroz, Guido Santos, Samuel Lima and Gustavo Carvalho, specialists at Delfos Energy, and presented at the Wind Europe 2025 Resource Assessment Symposium in Copenhagen.
To access the full study and rich content, fill in the form below:
Book a meeting
Let's connect and forge new partnerships
Custom Renewable Energy Solutions
Contact us today to discuss your renewable energy needs and find the perfect solution for your business.